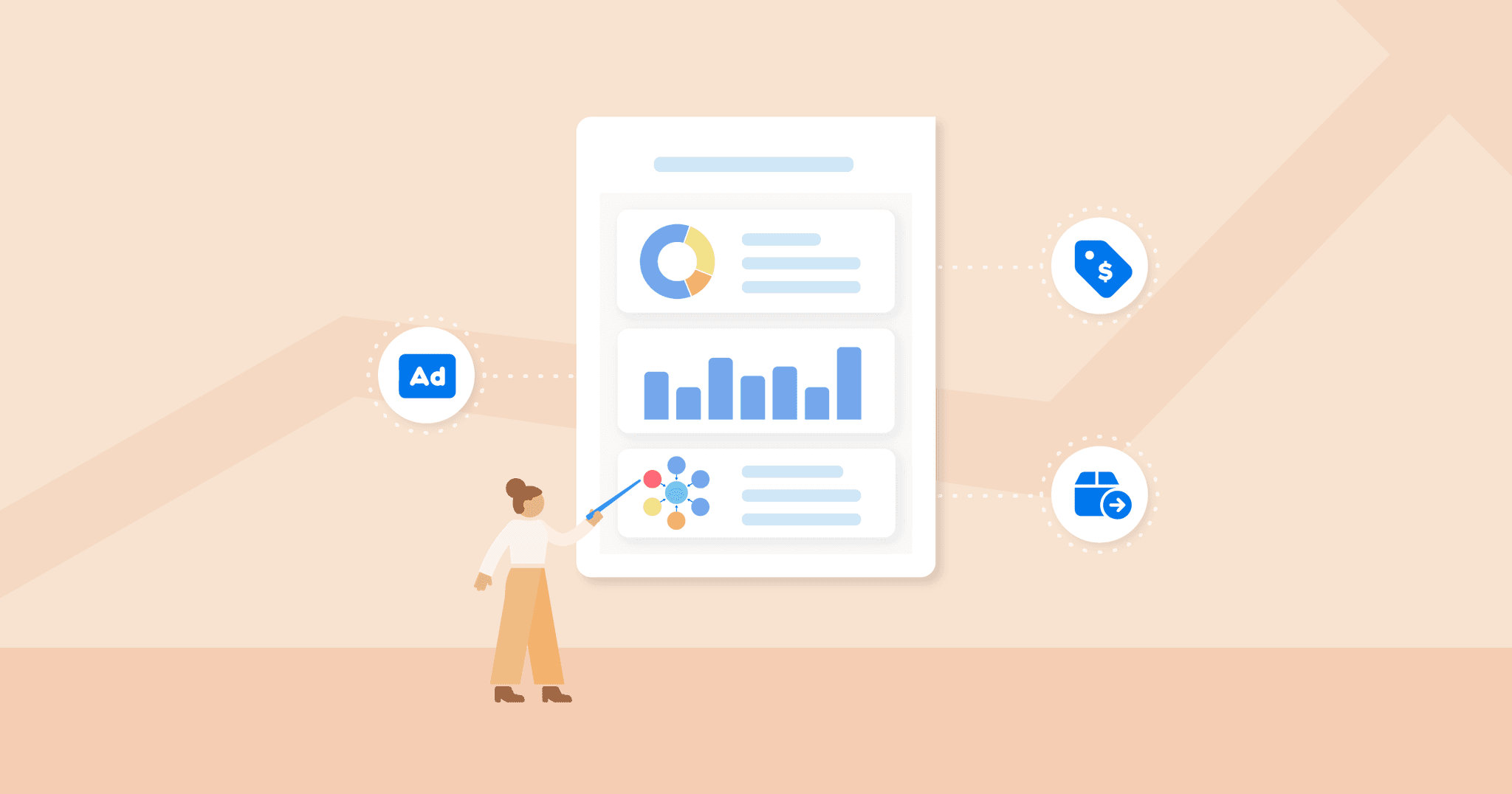
QUICK SUMMARY:
Media mix modeling is a statistical technique for analyzing the impact of diverse marketing and advertising efforts on sales and key performance indicators. It assesses historical data to determine the effectiveness of various channels like television, digital, and print. This article digs into media mix modeling, offering insights into evaluating the strengths and weaknesses of client campaigns, emphasizing the importance of clarity in marketing direction.
Understanding the complexities of modern marketing strategies is a game changer. One of the pivotal tools to achieve this is Media Mix Modeling, an analytical solution that assists in recognizing the impact of various marketing tactics across a variety of media channels.
Marketing is a sophisticated mix of multiple elements working in concert. Determining the influence of each individual tactic on a client's campaign–be it email marketing or SEO–can feel like navigating a labyrinth. With media mix modeling, it's as if you suddenly have a detailed map.
MMM takes all the threads of various marketing tactics–the clicks, the impressions, the conversions–and weaves them into a coherent narrative of success. It helps agencies understand where to direct marketing spend for maximum impact.
MMM is the process of quantifying and qualifying the effectiveness of different marketing tactics. It helps direct marketing spend in a manner that yields maximum results and helps agencies avoid the pitfalls of investing heavily in low-performing campaigns.
As we dive deeper into the nuances of Media Mix Modeling, you'll gain insights into the role it plays in illuminating the strengths and weaknesses of your client's campaigns. In the realm of marketing, the true advantage lies not just in the speed of execution but in the clarity of direction.
What Is Media Mix Modeling?
Media Mix Modeling is a predictive statistical analysis that maps the relationship between marketing efforts and sales outcomes.
This advanced method uses regression analysis to plot the relationship between one or more independent variables (e.g., ad spend, changes to pricing, seasonal incentives) and a dependent variable (e.g., sales, revenue, conversions).
Establishing the relationship between two or more variables is powerful–it means having a more predictive, scalable approach to sales outcomes. And what revenue or conversion-driven client doesn’t want that?
Media Mix Modeling Vs. Marketing Mix Modeling
In the marketing analytics arena, Media Mix Modeling (MMM) and Marketing Mix Modeling (also MMM) often find themselves at the center of many a discussion. While they might seem similar, considering they both have 'mix' and 'modeling' in their names (and let's face it, who doesn't love a good alliteration), they serve different, albeit interconnected, purposes.
Media Mix Modeling and Marketing Mix Modeling both quantify the impact of various marketing initiatives. They serve to improve the efficiency and effectiveness of marketing campaigns by offering insights into what's working and what needs a bit more elbow grease. Both of these models help optimize marketing spend, ensuring each dollar works as hard as possible.
Now, for the differences between a media mix model and a marketing mix model, and trust us, they're not as night and day as vampire bats and fluffy bunnies.
Media Mix Modeling, as the name suggests, focuses on different media channels. It seeks to understand the role each media type plays in driving the overall campaign performance. Whether it's digital, TV, radio, print, or any other form of media, the Media version of MMM aims to determine the contribution of each toward the marketing goals.
On the other hand, marketing mix models take a more holistic view. It doesn't just consider media channels but also factors in other marketing activities and elements like pricing strategies, product distribution, and even macroeconomic indicators. It's all about viewing the larger picture and determining how these varying elements interplay to impact the overall marketing performance.
While both models offer critical insights, they do so from different perspectives. Media Mix Modeling delves into the realm of media channels, while Marketing Mix Modeling takes into account a wider array of factors affecting marketing success.
Why Would Agencies Need a Media Mix Model?
Same story, different month: your agency needs a way to prove marketing ROI and differentiate itself from every other agency out there. So if you want to go a step above just presenting metrics, use the media mix modeling method to make accurate projections and smarter campaign decisions.
Media mix models serve as an analytical powerhouse, deciphering the enigma of marketing performance. They assess the degree to which each marketing strategy influences the outcome of a client's campaign.
Effective marketing is all about data storytelling. Your clients’ marketing campaigns don’t exist in silos, and neither should your data analysis. Without delving deeper, you’ll miss an opportunity for in-depth understanding and delivering spot-on recommendations.
From changes in ad spend to external marketing conditions, all these interconnected parts directly affect your clients’ sales outcomes. But if we’re being real, it isn’t the easiest or most straightforward relationship to understand (especially if we’re talking about big data sets).
Numbers aside, your enterprise-level clients’ sales and marketing teams also need to be on the same page. How often has the marketing team had to justify their campaigns or convince everyone they’re contributing to sales? It’s an uphill battle, and your clients look to your agency for a solution.
Using Media Mix Modeling means finding a goldmine in historical data and analyzing which marketing channels produce the highest ROI.
Consider the number of times your clients ask questions like:
What was the ROAS of our LinkedIn Ads spend for the past five years?
Should we reevaluate or reallocate our advertising spend to another channel to drive more sales?
Historically, which marketing campaigns have had the most significant impact on our sales outcomes?
What are the top-performing marketing channels for each geographic location (if your clients have multiple business locations)?
How would an increase of $X for Google Ads affect our number of conversions?
The Media Mix Modeling method equips your agency with historical insights to make future projections and tie in your efforts to your clients’ ROI–hello, client retention.
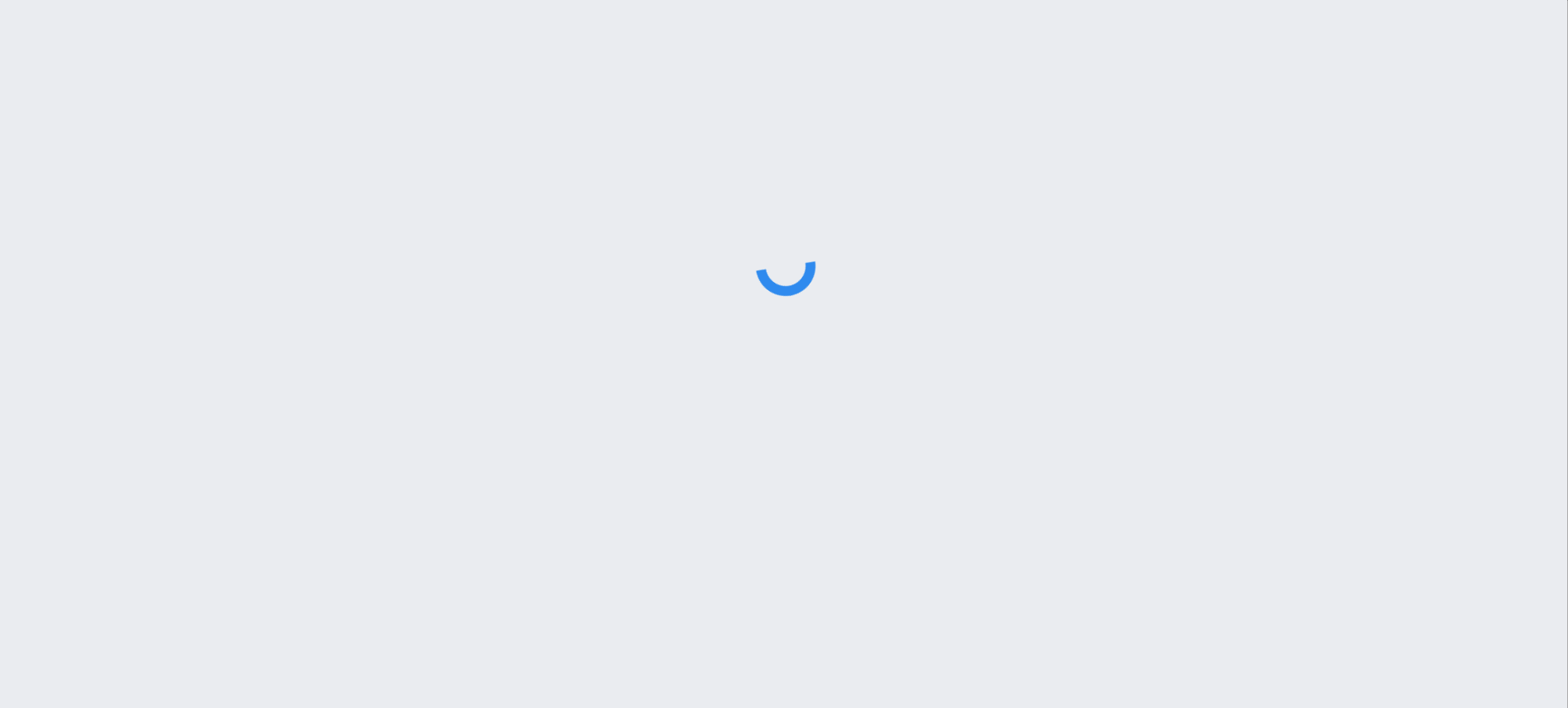
Create a solid data foundation from the get-go to retain your clients. Clearly communicate progress with visual dashboards, monitor trends, and perform data analysis–it’s free for 14 days on AgencyAnalytics.
Why Is Media Mix Modeling Important for Agencies?
Still on the fence about implementing media mix modeling? Here are a few reasons why it’s useful for agencies like you and how it differentiates your services from the rest.
1. Aggregates Big Data Sets and Pinpoints Historical Trends
This means:
Having data-driven insights to replicate marketing results under similar conditions
Predicting any ebbs or flows in sales outcomes (e.g., due to seasonal demands)
Relying on solid data trends to make recommendations
Accessing advanced data capabilities (which frees you from Google Data Studio limitations or buggy Excel formulas)
2. Creates Greater Alignment Between Sales and Marketing Teams
By uncovering exactly which marketing variables contribute to sales outcomes, your clients’ internal teams will have shared, laser-focused goals.
The result? More revenue and conversion potential. And a greater value is attributed to your agency–you’re directly helping improve their business without the extra work.
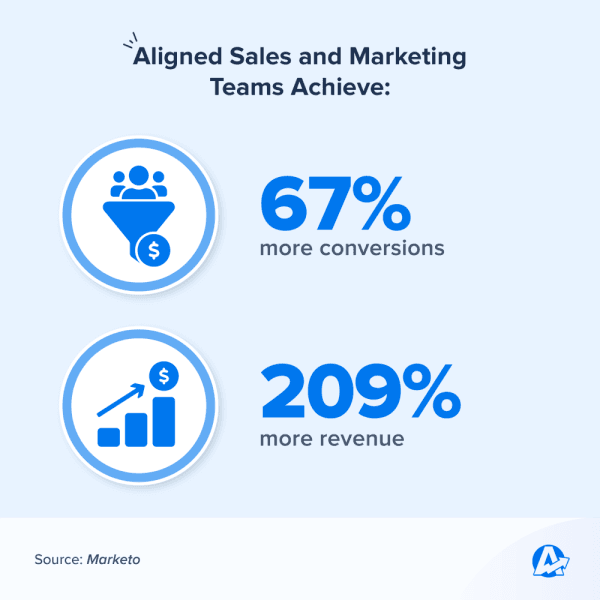
3. Factors in the Impact of External Conditions
Macroeconomic changes (e.g., a recession, rising inflation rates) are all things that should be considered when setting goals and KPIs or OKRs for the quarter.
Were your clients’ sales severely affected because of job layoffs in their target market? Perhaps rising living costs led to a dip in sales (even though their prices were competitive at the time). This big-picture data will add context to your clients’ business performances and explanations where needed.
4. Predicts How Changes in Marketing Variables Affect Sales Outcomes
Having the ability to forecast sales is powerful. It means reliable future projections and a solid basis for strategy revisions, which lets your clients strategize in advance.
In addition, your agency is able to determine the marketing mix that leads to the most favorable sales outcomes, which influences future campaigns. This means maximum revenue potential for your clients and more confidence in your agency’s capabilities.
How Media Mix Modeling Works
Let’s break down the different stages of the Media Mix Model.
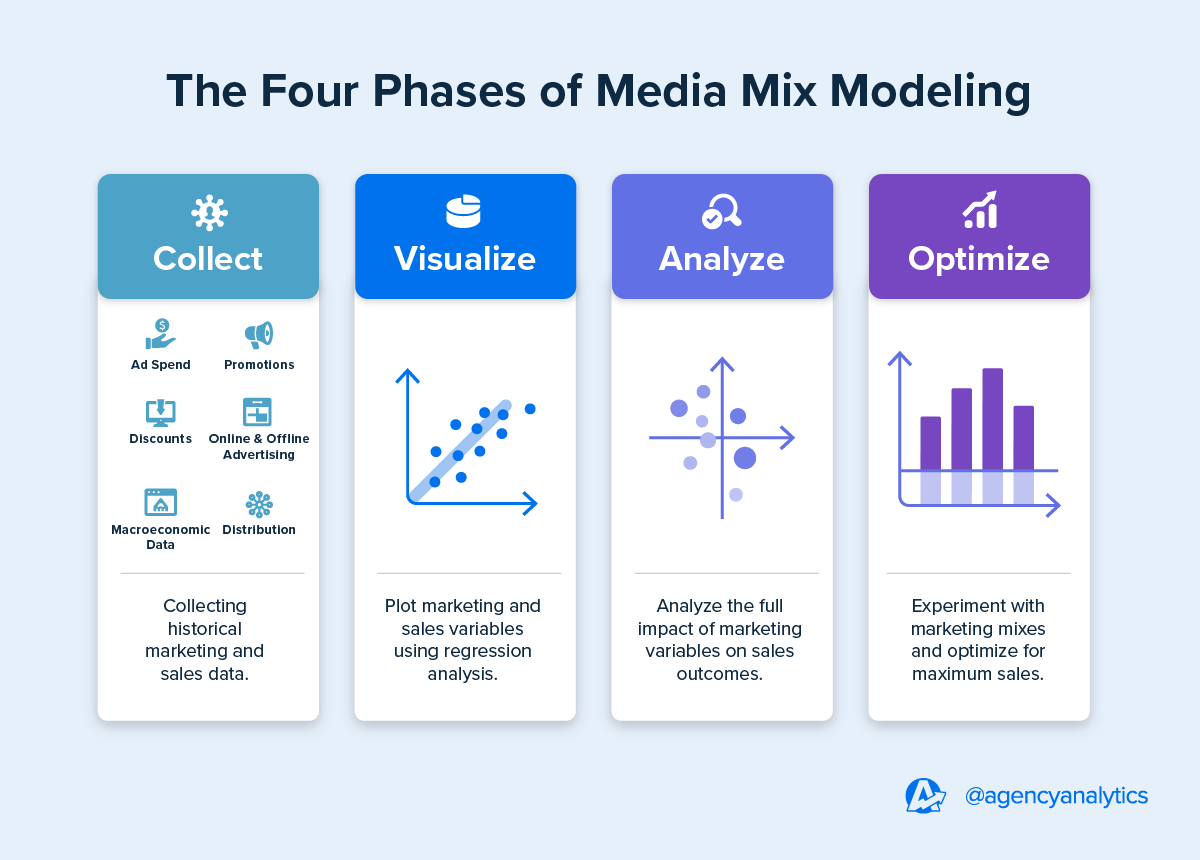
1. Data Collection
This stage involves collecting all the historical marketing and sales data used in the Media Mix Modeling exercise. This may include:
Sales and marketing ad spend across various distribution channels
Sales from promotional activities, discount incentives, and after pricing changes
Online advertising metrics
Offline marketing metrics (which may be limited or difficult to measure)
Macroeconomic variables (such as inflation rates)
Local-sensitive marketing data (if your clients have more than one business location)
Big data sets are required for this type of predictive modeling–at least two years’ worth. Additionally, this data should be well-formatted and optimized for whatever tool you use for Media Mix Modeling (e.g., Python, R, SAS).
2. Data Visualization (Modeling)
Next up, it’s time to choose the most appropriate predictive model. Different types of regression analyses are used in Media Mix Modeling, such as:
Linear Regression: This model plots the relationship between an independent marketing variable and a dependent sales variable. As the name implies, the result is a linear pattern. Multiple-linear regression also comes in handy to compare how different marketing variables affect sales outcomes
Non-linear Regression: In this predictive model, one or more independent marketing variable is mapped against one dependent sales variable. All variable relationships don’t follow a predictable pattern, and non-linear regression considers this for more complex marketing mixes
Time-series Regression: In this case, the relationship between one or more independent marketing variables and a dependent sales variable is mapped according to time intervals. This predictive model is beneficial for Media Mix Modeling, especially if there are seasonal ebbs and flows
Media Mix Modeling in Action
To help you understand this better, take the data visualization below (which is a non-linear regression). In this case, the independent variable is Facebook Ads spending, and the dependent variable is the number of leads.
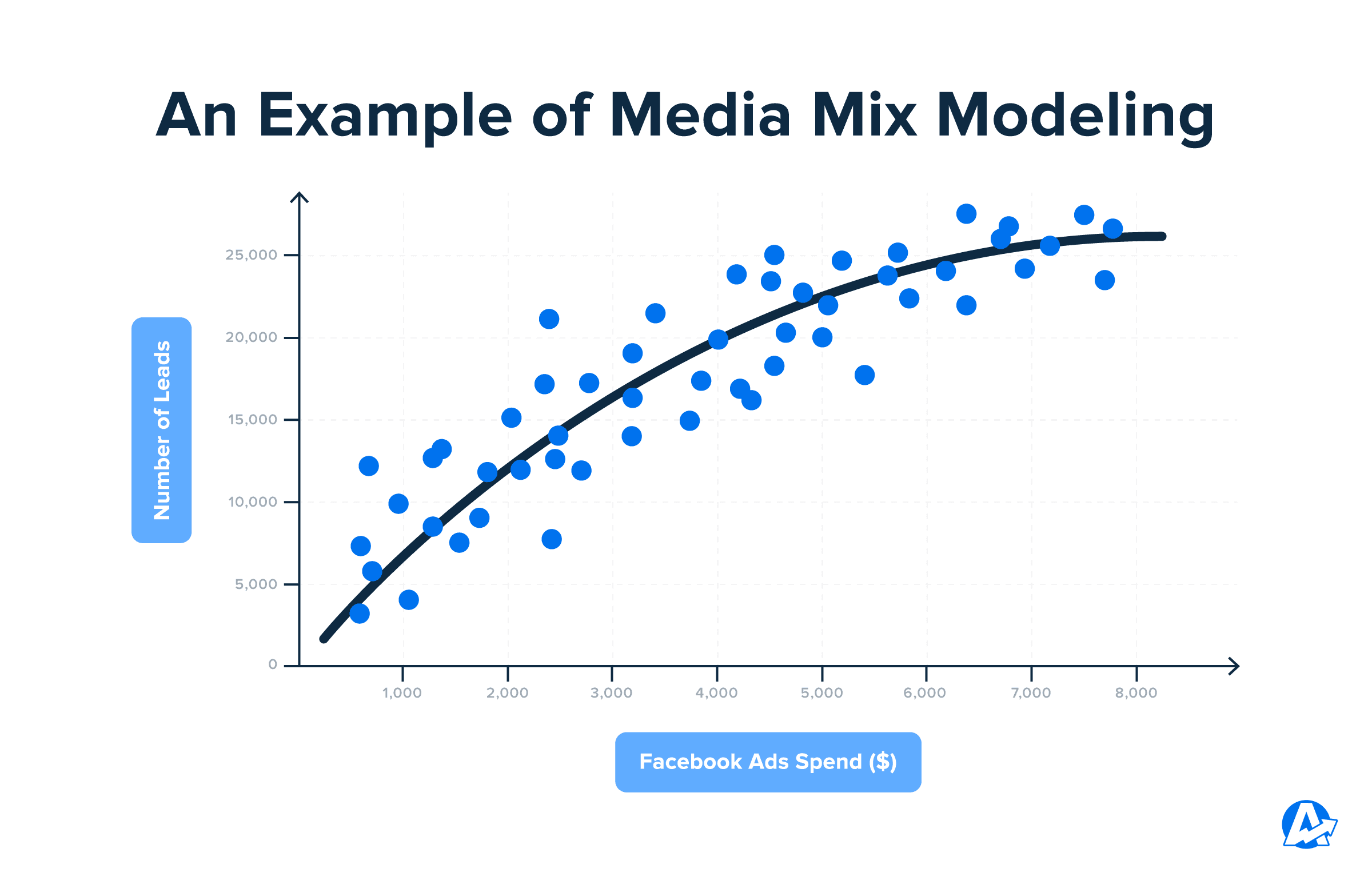
As seen from the graph, an increase in ad spend will lead to an uptick in the number of leads. But why exactly does this eventually level off instead of following a linear progression? Remember–even your clients’ most compelling ads will reach saturation over time.
So even as media spend and brand awareness increase, repeated exposure will lead to brand fatigue. As a result, viewers aren’t as inclined to take action (i.e., diminishing returns).
That’s why choosing a suitable predictive model goes a long way–a linear regression analysis wouldn’t have captured this theory.
3. Data Analysis & Insights
After you’ve run your regression analysis, it’s time to evaluate the results. To ensure you’re on the right track, ask the following questions:
Does the relationship between these variables make logical sense?
What was the main contributing factor to sales outcomes?
Is the inputted data rich enough to make reliable conclusions?
Do the data predictions seem realistic?
Are any of the predictions accurate for previous sales outcomes?
Are these results replicable for future marketing strategies?
Be sure to validate your chosen predictive model so it sets the proper foundation for your recommendations.
4. Media Mix Optimization
Once you’ve decided that your predictive model makes sense, use it to:
Create simulations of how different marketing inputs affect sales outcomes
Determine which marketing mix contributes to the best sales results
Inform media planning and any advertising adjustments
Align with your clients’ KPIs (such as marketing ROI, number of sales, number of website visits) and make future projections

No need to wait till a large-scale modeling exercise rolls around–keep on the pulse of your clients’ KPIs in real-time. Track data in a visually appealing way on AgencyAnalytics, free for 14 days.
Media Mix Modeling vs. Data-Driven Attribution
You may be wondering–how is Media Mix Modeling (MMM) different from marketing attribution models?
While they both provide insights and direction, there are a few differences to explore.
Similarities | Differences |
---|---|
Similarities Identify your clients’ top-performing marketing channels | Differences Marketing attribution models consider the customer journey whereas MMM doesn’t |
Similarities Establish a relationship between marketing efforts and sales outcomes | Differences Some marketing attribution models (such as multi-touch attribution) rely on third-party data; MMM doesn’t factor this in |
Similarities Are effective tools for allocating ad spends | Differences MMM focuses on long-term, big data; marketing attribution analyzes immediate metrics and user touchpoints |
Similarities Use data analysis methods to make deductions | Differences MMM uses regression analysis; marketing attribution models use machine learning technology |
It’s important to note that marketing attribution models are often built-in functionalities within marketing platforms (like Google Ads) and client reporting tools. Media Mix Modeling requires more heavy lifting, such as data export, modeling, and manipulation.
While they’re both distinct approaches, marketing attribution models help your clients understand customer touchpoints on a more granular level. On the flip side, use Media Mix Modeling for in-depth data analysis of historical data and making predictions.
How to Measure Media Mix Modeling
To grasp how media mix modeling works, here are the marketing metrics to measure and how they contribute to the overall picture of your client’s business health.
1. Sales Volume
In general terms, sales volume refers to the total number of sales for a given period. However, for more detailed data analysis, consider the following substages.
Base Sales
These are the sales that happen without injected ad spend or significant changes to your clients’ marketing strategies. Base sales are usually a result of:
Established, core product offerings or services that don’t require heavy advertising (e.g., well-known legacy brands).
Strong brand loyalty and repeat business (e.g., sales from subscription-based business models).
Keep in mind that while base sales are predictable over time, they may be affected by significant changes (e.g., the emergence of new, innovative competitors).
Incremental Sales
This term refers to any sales that happen as a result of any additional or supplementary marketing-related activities such as:
Introducing a new product offering or service
Offering discount incentives
Seasonal promotions
Offline and online paid advertising
As a quick reference, incremental sales are calculated by the formula below:
Incremental Sales = Total Sales - Base Sales
But why exactly is this distinction needed? Let’s suppose your client injected an additional $1,000 into Facebook Ads and ended up with $10,000 in total sales on a spend of $2,000. From the looks of it, this digital marketing campaign was successful. (After all, there’s a significant marketing ROI).
However, what if your client’s base sales were already $9,500? That would mean their incremental sales were significantly less ($500 to be exact) and much lower ROI. As a result, you may consider investing in other digital marketing channels.
By breaking down sales volume this way, you’ll understand the exact marketing ROI without established brand equity.
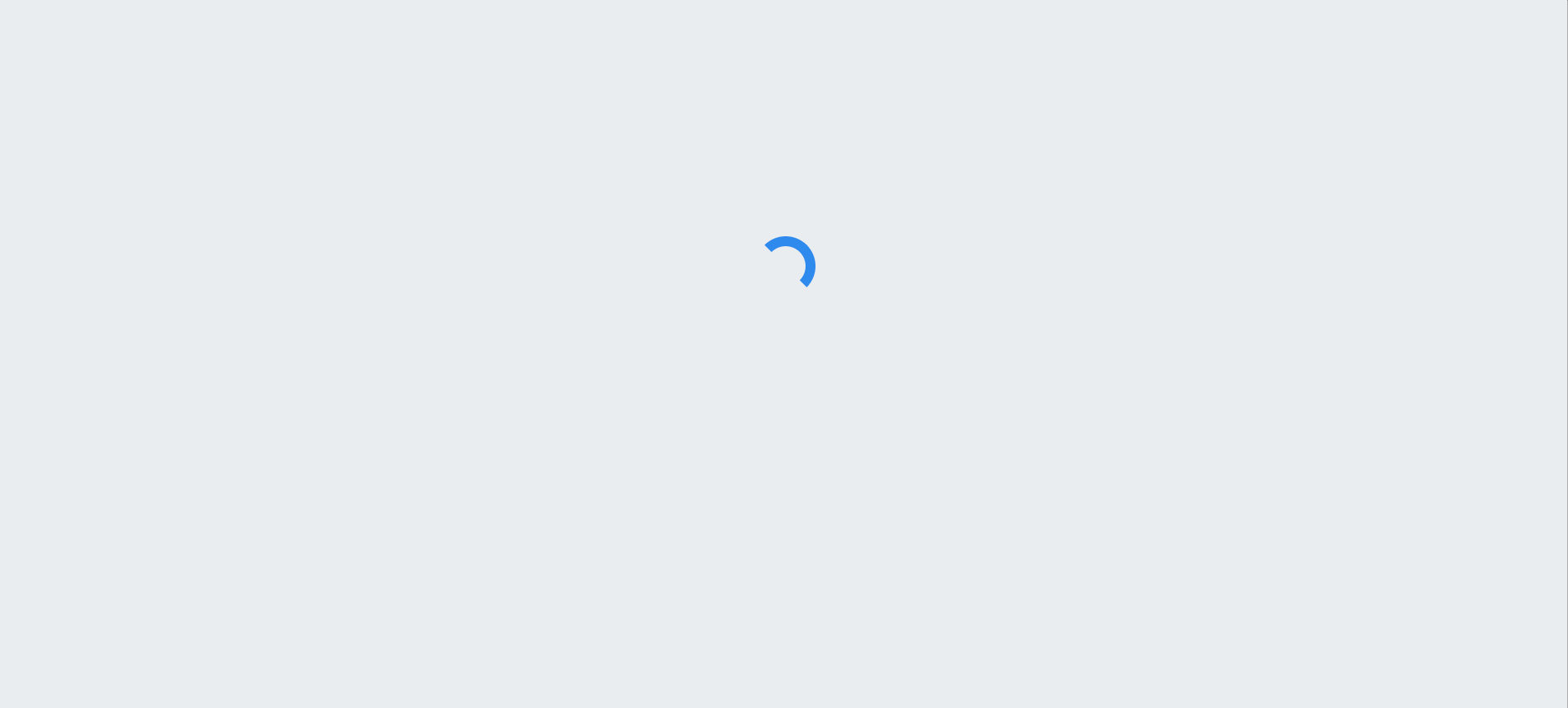
Put a system in place to collate data in a scalable way. See how your clients’ marketing channels perform over time–try it on AgencyAnalytics, free for 14 days.
2. Media and Advertising
Your agency knows all too well that not all marketing and advertising campaigns are built equally. From large-scale programmatic advertising to offline activities (like print ads and billboards), different campaigns will result in different sales outcomes.
And as your clients’ trusted marketing agency, how will you decide where to invest their ad spend, or even which ad types are most effective? To answer their questions, use Media Mix Modeling to:
Determine an optimal ad type (e.g., historically, a client’s Instagram Ads may have had more traction than Twitter Ads).
Decide on the best advertising parameters (e.g., the most optimal TV commercial length based on past data).
3. Distribution
When it comes to getting your clients’ messages out there, evaluating distribution channels is crucial. While digital is undoubtedly more mainstream nowadays, don’t overlook the power of other routes.
For example, should your clients rely solely on social media channels for advertising? Perhaps a relatively significant share of their target audience can be reached by more traditional media forms (like TV or radio) and mobile apps. And who knows–maybe a combination of distribution channels will lead to the highest sales outcomes.
Implementing the Media Mix Model means looking at the sales volume generated by each distribution channel and strategizing accordingly. Make sure to include all the aggregated data points needed, including digital marketing analytics, sales data, and call tracking (if applicable).
The Limitations of Media Mix Modeling
Although Media Mix Modeling is a powerful tool for data analysis, it isn’t perfect–here are a few limitations to consider below.
Media Mix Modeling needs big data sets to make deductions and track historical trends. If your clients are now starting off with data collection or have significant gaps in data, media mix modeling isn’t the right fit (at least for now).
There may be implied correlations in the absence of significant dependencies between variables. While your regression analysis may have resulted in a graphical relationship, it doesn’t necessarily mean anything if there isn’t any logical basis for it (which is why your own data analysis is needed).
It's a complex and advanced technique. Let’s face it–media mix modeling isn’t a simple procedure to run. It’s an in-depth data analysis that requires expertise, additional training for your staff, and significant capital from your clients.
Media Mix Modeling doesn't consider the customer journey on a micro-level. While Media Mix Modeling is certainly useful for big-picture marketing strategies, it isn’t a reliable technique for understanding customer behavior or decisions.
Don’t let these limitations deter you, though! Use Media Mix Modeling where appropriate and pitch it to your clients who will benefit from robust data analysis.
Arm Your Agency With Powerful Media Mix Modeling Insights
Media Mix Modeling is a dynamic approach to seamlessly blend data and uncover your clients’ most valuable marketing channels. Knowledge is power, and reliable data analysis is just what your agency needs to make informed decisions before making recommendations.
But to even consider Media Mix Modeling, your agency needs the right infrastructure in place to house your clients’ marketing metrics reliably and consistently over time. The last thing you want is gaps in your clients’ data or realizing they don’t even qualify for an exercise like Media Mix Modeling.
No need to scramble for data across multiple marketing platforms–use AgencyAnalytics to streamline insights in one place.
All you have to do is download the AgencyAnalytics for Google Sheets app (currently in beta) and export their data into a spreadsheet–it’s that simple. So when that 2-year mark rolls around, it’ll be much easier to package your clients’ big data sets into an appropriate format for Media Mix Modeling.
Set your enterprise-level clients up for success! From easy-to-understand visual dashboards to automated data retrieval, AgencyAnalytics is built for agencies like you.
Consolidate your clients’ metrics in a centralized place. Think long-term and invest in a sustainable reporting solution like AgencyAnalytics–sign up for a free 14-day trial today!
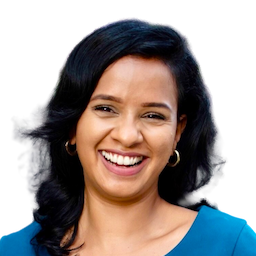
Written by
Faryal Khan is an experienced digital marketer and brand photographer with 9+ years of experience. With a passion for content creation, she creates value through editorial storytelling, data-driven insights, and captivating visuals.
Read more posts by Faryal Khan ›Get Started for Free
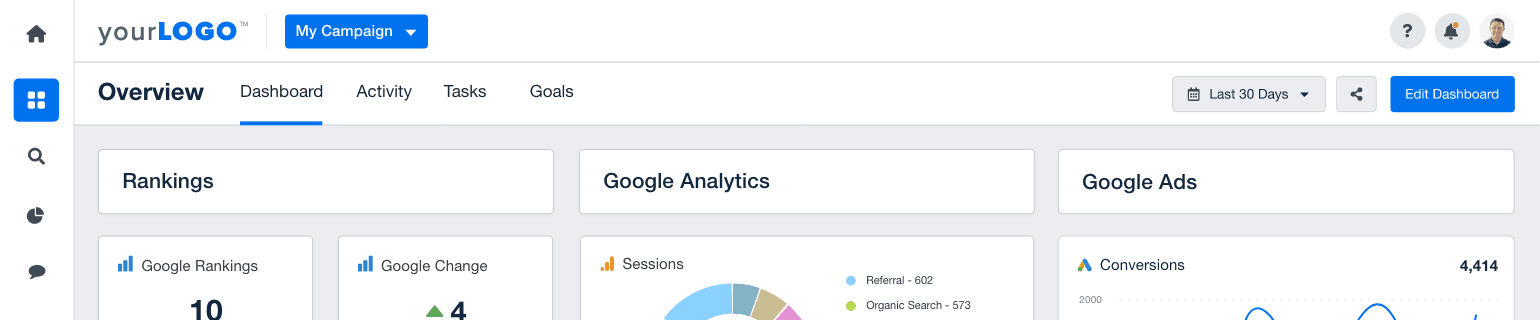